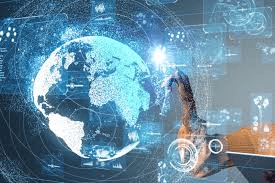
This article is written by Ridhi Aggarwal of 4th Semester of Kirit P. Mehta school of law, NMIMS, an intern under Legal Vidhiya
Abstract
The integration of artificial intelligence (AI) and automation is revolutionizing corporate governance, enhancing decision-making and operational efficiency while introducing challenges related to accountability, transparency, and ethics. Current frameworks often fail to address the complexities of AI-driven technologies, such as liability for autonomous decisions, algorithmic bias, and regulatory compliance. This paper examines these gaps and proposes solutions, including AI-specific governance codes, improved accountability mechanisms, and the integration of ethical considerations into corporate policies. A comparative analysis of international legal practices highlights best practices and emphasizes the need for global collaboration to harmonize AI governance laws and address cross-border challenges. The study aims to bridge the gap between technology and governance, offering insights for policymakers, corporate leaders, and researchers to build robust legal frameworks that promote responsible AI adoption while protecting stakeholder interests.
Keywords
Corporate Governance, Artificial Intelligence, Automation, Legal Frameworks, Accountability, Ethics
Introduction
Corporate governance ensures accountability, transparency, and ethical decision-making within businesses. The rise of artificial intelligence (AI) and automation is transforming governance structures, enhancing efficiency but also raising legal and ethical concerns. AI technologies like predictive analytics and natural language processing offer significant benefits, such as improved risk management and streamlined operations. However, their adoption introduces challenges around accountability, transparency, and ethics, necessitating a re-evaluation of existing governance frameworks to address the complexities of AI.
The integration of AI and automation into corporate governance presents a host of legal and regulatory challenges. Traditional governance models and legal frameworks often fall short in addressing the unique characteristics of AI systems, such as their ability to make autonomous decisions and learn through machine learning algorithms. This regulatory gap creates uncertainty when it comes to determining responsibility for decisions and outcomes influenced by AI. The situation becomes particularly complex in scenarios involving unintended consequences or system failures[1], where pinpointing accountability can be challenging.
A significant challenge arises from the opacity of AI algorithms, commonly referred to as the “black box” problem. Many AI systems operate in ways that are difficult for even their developers to fully understand, making it hard for stakeholders to ensure transparency and accountability. This lack of clarity poses ethical dilemmas, as it becomes unclear who should be held responsible for AI-driven decisions. Furthermore, the potential for bias in AI systems complicates matters, as such biases could result in unfair or discriminatory outcomes, exposing organizations to reputational and legal risks.[2]
Given these complexities, corporations face the critical task of balancing innovation with ethical obligations and regulatory compliance. The adoption of AI and automation requires the development of governance frameworks that prioritize transparency, establish clear lines of accountability, and ensure adherence to ethical standards. By proactively addressing these issues, organizations can harness the benefits of AI while maintaining public trust and meeting their legal and social responsibilities.[3]
The Role of Artificial Intelligence and Automation in Corporate Governance
Artificial intelligence (AI) and automation are transforming the landscape of corporate governance by reshaping how organizations make decisions, manage operations, and ensure compliance with regulations. These technologies have introduced a new era of efficiency and precision, enabling businesses to make strategic decisions supported by data-driven insights that were previously unattainable through conventional methods.
AI-powered systems are increasingly being integrated into boardrooms to facilitate strategic decision-making. By leveraging advanced analytics, corporations can process vast amounts of data, uncover patterns, and predict outcomes with unprecedented accuracy. This capability allows decision-makers to base their strategies on robust evidence, fostering more informed and effective governance practices.[4]
Automation, on the other hand, is revolutionizing operational workflows by streamlining repetitive tasks, minimizing human errors, and ensuring consistent outcomes. For example, automated systems are particularly effective in managing compliance monitoring and reporting activities. These systems can rapidly adapt to changes in legal and regulatory frameworks, ensuring that organizations meet their obligations with greater accuracy and speed. [5]This is especially critical in highly regulated industries where non-compliance can result in significant penalties and reputational harm.
However, the integration of AI and automation into corporate governance is accompanied by a range of challenges. One of the most pressing concerns is the lack of transparency in AI algorithms, often referred to as the “black box” issue. When AI systems make decisions, it can be difficult to trace the logic or rationale behind those decisions, raising questions about accountability. This lack of clarity creates ethical and legal dilemmas, particularly when adverse outcomes occur. Additionally, biases embedded in AI algorithms can lead to discriminatory practices, potentially exposing companies to legal risks and damaging their reputation.
Despite these obstacles, the benefits of incorporating AI and automation into corporate governance are substantial. These technologies enhance operational efficiency, reduce costs, and improve compliance with regulatory standards. However, their adoption necessitates a proactive approach to addressing the ethical and legal implications. Corporations must ensure that AI systems align with established governance principles, uphold transparency, and reflect societal values. By implementing robust oversight mechanisms and promoting ethical practices, businesses can harness the potential of AI and automation while mitigating associated risks.
Therefore , AI and automation are poised to play a pivotal role in shaping the future of corporate governance. While they present significant opportunities for enhancing decision-making, operational efficiency, and compliance, addressing their challenges is crucial to maximizing their benefits responsibly.
Legal Challenges in Governing AI and Automation
The integration of artificial intelligence (AI) and automation into corporate governance frameworks presents a range of intricate legal challenges that demand careful consideration. One of the most significant issues revolves around accountability and liability. When AI systems are employed to make decisions, determining responsibility for the outcomes becomes complex, especially in cases where errors, unintended consequences, or malfunctions occur. Traditional corporate governance structures, which are built on the premise of human decision-making, are ill-equipped to address scenarios where decisions are autonomously made by algorithms. [6]This creates an ambiguous legal Gray area regarding who should bear responsibility—the AI developers who designed the system, the corporations that deploy and rely on it, or the individuals responsible for overseeing its implementation and operation.
Another critical challenge is ensuring compliance with existing regulatory frameworks. Most current laws and regulations were developed before the advent of AI technologies and do not account for their unique characteristics, such as the adaptive and self-learning nature of machine learning algorithms. As a result, it can be difficult to guarantee that AI-driven systems operate within the bounds of legal and regulatory standards, particularly in highly regulated sectors like finance, healthcare, and manufacturing.[7] These industries often require strict adherence to compliance rules, and the evolving nature of AI adds an additional layer of complexity. Furthermore, the lack of a unified global approach to AI governance exacerbates these issues, creating inconsistencies across jurisdictions. Multinational corporations face significant challenges in navigating conflicting legal requirements and regulatory expectations in different countries.
Ethical and social implications further complicate the legal landscape surrounding AI and automation. One of the primary concerns is the potential for AI systems to perpetuate biases embedded in their training data. When such biases influence decision-making processes, they can lead to discriminatory practices that violate anti-discrimination laws and undermine corporate commitments to social responsibility. [8]Additionally, the opacity of many AI algorithms, often referred to as the “black box” problem, raises serious concerns about transparency and fairness. The inability to fully understand or explain how AI systems arrive at decisions makes it challenging to assess their compliance with both legal standards and ethical norms.[9] This lack of transparency can undermine stakeholder trust and expose organizations to legal liabilities.
Balancing innovation with the need to protect societal interests poses a critical legal and ethical challenge. While AI and automation offer significant potential to enhance efficiency and decision-making, their integration into corporate governance must be guided by robust frameworks that prioritize accountability, transparency, and fairness. Addressing these challenges requires ongoing collaboration between policymakers, corporations, and technology developers to establish clear legal standards and ethical guidelines that support responsible innovation while safeguarding public trust.
Current Legal Frameworks and Gaps
The legal frameworks currently governing artificial intelligence (AI) and automation in corporate governance are largely rooted in traditional laws that struggle to address the unique challenges posed by these transformative technologies. Most corporate governance regulations are designed with human decision-makers in mind, emphasizing principles such as fiduciary duties, accountability, and compliance that assume human oversight and responsibility.[10] However, these frameworks offer little guidance on the integration of AI-driven systems, leaving a significant gap in defining the roles and responsibilities of AI systems and their developers. For instance, while fiduciary duties focus on the accountability of board members and executives, they do not account for scenarios where critical decisions are autonomously made by algorithms. [11]This creates uncertainty about liability in cases of errors, unintended consequences, or system failures stemming from AI-driven decision-making.
Some existing regulations, such as the General Data Protection Regulation (GDPR) in Europe, partially address issues related to automation, particularly in areas like data privacy and algorithmic transparency. The GDPR mandates certain levels of transparency and accountability in automated processes, such as the right of individuals to understand and contest decisions made by AI systems. [12]However, these provisions are limited in scope and do not extend to broader corporate governance issues, such as determining liability for decisions made by autonomous systems or setting standards for ethical AI deployment. This regulatory gap leaves organizations without clear guidance on how to align AI integration with their governance obligations.
Another critical shortcoming in the current legal landscape is the absence of standardized guidelines for algorithmic accountability and the ethical use of AI. While several countries and international organizations have developed ethical guidelines for AI, these frameworks are often non-binding and lack mechanisms for enforcement. [13]This limits their effectiveness in driving responsible AI practices. Additionally, antitrust and competition laws are ill-equipped to address the monopolistic tendencies of AI-driven platforms, which often accumulate vast amounts of data and market influence.[14] These monopolistic practices can lead to market distortions, stifling innovation and competition. Without updated regulations to address these dynamics, corporations using AI may inadvertently contribute to unfair market practices.
The lack of a unified global framework further complicates the governance of AI and automation. Corporations operating across multiple jurisdictions face inconsistent legal requirements, leading to compliance challenges and opportunities for regulatory arbitrage. [15]This fragmentation in legal standards hinders the development of cohesive strategies for managing AI’s impact on governance, increasing the risk of uneven application and enforcement of ethical and legal norms.
Therefore, the current legal frameworks inadequately address the complexities introduced by AI and automation in corporate governance. This regulatory inadequacy highlights the urgent need for updated, AI-specific laws that incorporate principles of transparency, accountability, and ethical responsibility. Additionally, harmonizing international standards is essential to ensure consistent application across jurisdictions, fostering a more cohesive and effective approach to governing AI technologies in a globalized business environment.
Proposals for Enhanced Corporate Governance
The swift integration of artificial intelligence (AI) and automation into corporate environments necessitates a proactive and comprehensive approach to governance. As these technologies reshape decision-making, operations, and compliance mechanisms, corporations must adopt forward-looking strategies to address their unique challenges. The following proposals outline key measures for enhancing corporate governance in this transformative era.
- Development of AI-Specific Governance Codes
To address the distinct complexities of AI and automation, there is an urgent need for governance codes tailored specifically to these technologies. These codes should be built on core principles of transparency, accountability, and fairness. Transparency entails that corporations disclose how their AI systems are designed, trained, and deployed, including the datasets and algorithms used. Such disclosure is essential for stakeholders to evaluate the integrity and reliability of AI systems.[16] Additionally, these governance codes must mandate robust mechanisms for algorithmic accountability. This includes creating frameworks that allow organizations to trace the decision-making processes of AI systems, enabling them to identify responsibility in cases of errors, unintended outcomes, or harm. For instance, incorporating explainable AI (XAI) technologies can provide insights into how and why an AI system arrived at a particular decision. [17]Regulatory requirements should also compel organizations to conduct regular impact assessments and establish clear accountability structures to manage risks associated with AI deployment. - Ethical Governance Models
Embedding ethical considerations into corporate governance structures is another critical step. Corporations must prioritize ethical AI practices by incorporating guidelines that focus on mitigating biases, protecting individual privacy, and upholding human rights[18]. These ethical principles should be integrated into corporate charters, forming the foundation of an organization’s approach to AI and automation. To ensure adherence to these principles, companies can establish ethical review boards tasked with evaluating AI systems and their potential impact on society. These boards should include diverse stakeholders, including technologists, ethicists, and representatives from affected communities, to provide comprehensive oversight. Independent audits of AI systems can further strengthen accountability by verifying compliance with ethical and legal standards. [19]By institutionalizing these practices, organizations can build trust with stakeholders while minimizing risks associated with unethical AI use. - Enhanced Stakeholder Engagement
A collaborative approach to governance is essential in navigating the societal impacts of AI and automation. Corporations must actively involve a wide range of stakeholders, including employees, customers, policymakers, and community representatives, in the development and implementation of AI policies. Engaging stakeholders not only promotes transparency but also ensures that diverse perspectives inform corporate strategies, leading to more equitable and socially responsible outcomes.[20] Mechanisms for stakeholder engagement may include public consultations, advisory panels, and regular reporting on AI governance practices. By fostering dialogue and collaboration, corporations can align their AI strategies with societal values and expectations, reducing the risk of reputational damage and fostering greater public trust. - International Collaboration and Harmonization of Standards
Given the global nature of AI and automation, international collaboration is critical to address the challenges of cross-border operations and regulatory inconsistencies. Policymakers and industry leaders must work together to establish cohesive international standards and frameworks for AI governance.[21] This can be achieved through treaties, agreements, or multilateral initiatives that promote uniform principles of transparency, accountability, and ethical responsibility.
The integration of AI and automation into corporate governance demands innovative and proactive measures to ensure responsible and ethical use of these technologies. By developing AI-specific governance codes, embedding ethical principles into corporate frameworks, enhancing stakeholder engagement, and fostering international collaboration, corporations can navigate the challenges and opportunities presented by AI and automation. These proposals aim to strike a balance between innovation and accountability, ensuring that technological advancements contribute to sustainable and equitable corporate practices in a rapidly evolving global landscape.
Case Studies
Successful Applications of AI Governance
Several corporations have demonstrated leadership in implementing robust AI governance frameworks, setting industry benchmarks for responsible and ethical use of AI.
- Microsoft’s AI Governance Framework[22]
Microsoft has been a trailblazer in establishing comprehensive AI governance practices. The company’s efforts are anchored in its AI ethics initiative, which focuses on six core principles: fairness, reliability and safety, privacy and security, inclusiveness, transparency, and accountability. Microsoft has institutionalized these principles through the creation of an AI ethics committee called the AI, Ethics, and Effects in Engineering and Research (Aether) Committee.
This committee evaluates potential ethical implications of AI projects, ensuring that technologies align with societal and corporate values. For example, Microsoft’s commitment to algorithmic fairness seeks to identify and mitigate biases in AI systems, promoting equitable outcomes. The company also prioritizes stakeholder consultation, involving employees, customers, and external experts in shaping AI policies. By embedding these principles into its operational framework, Microsoft has set a high standard for AI governance, providing a replicable model for other organizations.
- Google’s AI Principles[23]
Google has adopted a similarly proactive approach to AI governance through the implementation of its AI Principles. These principles include commitments to develop AI that is socially beneficial, avoid harm, and uphold ethical standards. For instance, Google has explicitly stated that it will not design or deploy AI technologies for purposes that violate human rights or facilitate mass surveillance.
The company has also established internal mechanisms for ethical oversight, including advisory panels and independent audits. Google’s decision to focus on transparency is evident in its efforts to explain AI functionalities and provide users with control over AI-powered tools. This approach not only enhances public trust but also demonstrates the potential of corporate governance to address societal concerns about AI.
Failures and Lessons Learned
While successful examples highlight best practices, failures in AI governance underscore the consequences of inadequate oversight and the urgent need for regulatory and ethical safeguards.
- Cambridge Analytica Scandal[24]
The Cambridge Analytica case is a stark example of governance failure involving AI-driven data analytics. The company used AI algorithms to harvest and analyze personal data from millions of Facebook users without their consent. This data was then exploited to influence political campaigns, raising significant ethical and legal concerns.
The public backlash from this incident led to widespread criticism of both Cambridge Analytica and Facebook, highlighting the risks of insufficient oversight in managing AI technologies. This case exposed glaring gaps in corporate governance, particularly around data privacy and accountability. It also underscored the need for stringent regulatory frameworks to prevent the misuse of AI and ensure that companies adhere to ethical practices. The fallout from this scandal resulted in heightened scrutiny of data practices and prompted legislative action, such as the strengthening of data protection laws like the GDPR.
- Boeing 737 MAX Crisis[25]
The Boeing 737 MAX crisis offers another cautionary tale about the dangers of governance failures in automation. A series of fatal crashes involving the 737 MAX aircraft were linked to the malfunctioning of the Maneuvering Characteristics Augmentation System (MCAS), an automated control system designed to enhance flight safety. Investigations revealed that inadequate testing, poor communication, and insufficient pilot training contributed to the disaster.
This crisis exposed critical gaps in accountability and oversight. While automation was intended to improve operational efficiency, the lack of robust governance measures to evaluate the system’s reliability led to catastrophic consequences. The incident highlighted the urgent need for regulatory reforms to govern the use of advanced technologies in critical systems. It also emphasized the importance of transparency, rigorous testing, and comprehensive training as essential components of AI and automation governance.
These examples collectively reinforce the importance of adopting comprehensive, transparent, and ethically grounded governance practices to ensure that AI and automation serve societal interests without compromising safety, privacy, or public trust. As AI continues to evolve, learning from these case studies will be instrumental in shaping the future of corporate governance.
Future Directions
The rapid evolution of artificial intelligence (AI) and automation is transforming corporate governance, reshaping decision-making, compliance, and operational structures. Future strategies must address these changes through research, innovation, and policy development in key areas:
- AI and Corporate Culture
AI reshapes organizational dynamics, impacting leadership, decision-making, and employee roles. While it can enhance efficiency and creativity by automating tasks, it may also lower morale and trust if not managed inclusively. Research is needed to harmonize human and AI contributions and foster ethical corporate cultures.
- Mitigating Unintended Consequences
AI systems risk reinforcing biases or exacerbating inequalities. Organizations must adopt fairness metrics, bias-detection tools, and regular audits. Interdisciplinary collaboration among technologists, ethicists, and sociologists is crucial to mitigate these risks and understand AI’s broader societal impact.
- Innovations in Governance
Emerging technologies like explainable AI (XAI), decentralized autonomous organizations (DAOs), and machine learning compliance tools demand updated governance models. XAI enhances transparency and trust, DAOs challenge centralized control, and AI compliance tools improve regulatory adherence, requiring robust oversight frameworks.
- Legal and Regulatory Frameworks
Adaptive legal frameworks are essential for addressing AI’s unique challenges, including liability, transparency, and ethics. Regulatory sandboxes can balance innovation with oversight, helping policymakers craft responsive and forward-looking laws.
- Interdisciplinary and Global Collaboration
Governance must involve experts from law, technology, and ethics to balance innovation with societal needs. International collaboration is vital for harmonizing AI standards, addressing regulatory inconsistencies, and ensuring equitable global benefits through shared knowledge and capacity-building initiatives.
By addressing these challenges, corporations and policymakers can responsibly leverage AI for sustainable and equitable growth.
Conclusion
As AI and automation redefine corporate governance, their integration presents both opportunities and significant challenges. AI can enhance governance by improving decision-making accuracy, reducing human error, and enabling data-driven strategies. However, this comes with critical concerns that traditional governance models struggle to address, such as algorithmic bias, opacity (the “black box” problem), liability for autonomous decisions, and broader ethical implications.
AI systems raise fundamental questions about accountability, especially when algorithms make autonomous decisions. Determining legal and ethical responsibility becomes complex when errors cause financial loss, reputational damage, or harm. Current legal frameworks assume human oversight, leaving gaps where decision-making authority is vested in AI. Algorithmic bias further complicates this, as biased data can lead to discriminatory outcomes, violating equality laws and creating legal liabilities. Transparency is another key issue; without clear mechanisms to explain AI decisions, stakeholder trust may erode, and regulatory compliance becomes challenging.
To address these challenges, AI-specific governance codes are essential, establishing guidelines for transparency, accountability, and fairness in AI deployment. These codes should ensure that corporations disclose the design, training, and decision-making processes of AI systems. Additionally, fostering international collaboration is crucial for creating harmonized regulatory standards that allow multinational corporations to navigate cross-border compliance more effectively.
Finally, AI integration into corporate governance must align with societal values. Policymakers and corporations should prioritize stakeholder trust by embedding ethical review processes, conducting regular audits, and ensuring that AI systems uphold human rights and protect the public interest. This proactive approach is vital to ensuring that AI enhances corporate governance while mitigating risks and safeguarding accountability, equity, and public trust.
References
- https://www.tandfonline.com/doi/full/10.1080/10383441.2024.2405752?utm
- https://www.researchgate.net/publication/353034683_Corporate_Governance_of_Artificial_Intelligence_in_the_Public_Interest
- https://scholarship.law.edu/cgi/viewcontent.cgi?article=3646&context=lawreview&utm
- https://virtusinterpress.org/IMG/pdf/clgrv6i3p12.pdf?utm
- https://www.researchgate.net/publication/382497179_The_Impact_of_Artificial_Intelligence_on_Corporate_Governance
- https://link.springer.com/article/10.1007/s10997-020-09519-9?utm
- https://digitalcommons.law.seattleu.edu/cgi/viewcontent.cgi?article=2886&context=sulr&utm
[1] Göktürk Kalkan, The Impact of Artificial Intelligence on Corporate Governance, 18 J. Corp. Fin. Res. 17 (2024)
[2] The AI Governance Challenge, S&P Global
[3] The Role of Corporate Governance in Responsible AI, Article One Advisors
[4] The Corporate Governance Institute, AI and Boardroom Decision-Making,.
[5] Wall Street Journal, AI Can Take the Slog Out of Compliance Work, but Executives Not Ready to Fully Trust It.
[6] Luciano Floridi & Josh Cowls, A Unified Framework of Five Principles for AI in Society, 1 Harv. Data Sci. Rev. (2019).
[7] Sandra Wachter, Brent Mittelstadt & Luciano Floridi, Why a Right to Explanation of Automated Decision-Making Does Not Exist in the General Data Protection Regulation, 7 Int’l Data Privacy L. 76 (2017).
[8] Ryan Binns, Fairness in Machine Learning: Lessons from Political Philosophy, in Proceedings of the 2018 Conference on Fairness, Accountability, and Transparency 149 (2018)
[9] Mittelstadt, B. D., Allo, P., Taddeo, M., Wachter, S., & Floridi, L. (2016). The ethics of algorithms: Mapping the debate. Big Data & Society, 3(2).
[10] Fenwick, Mark, Joseph A. McCahery & Erik P. M. Vermeulen, The End of “Corporate” Governance: Hello “Platform” Governance, 20 Eur. Bus. Org. L. Rev. 171 (2019)
[11] Chengyi Huang & Stein Johansen, AI and Corporate Governance: Shaping the Future of Decision-Making, 35 AI & Soc’y 723 (2020).
[12] Regulation (EU) 2016/679 of the European Parliament and of the Council of 27 April 2016 on the Protection of Natural Persons with Regard to the Processing of Personal Data and on the Free Movement of Such Data (General Data Protection Regulation), arts. 13–15, 2016 O.J. (L 119) 1.
[13] Luciano Floridi, Josh Cowls, Thomas C. King & Mariarosaria Taddeo, How to Design AI for Social Good: Seven Essential Factors, 24 Sci. & Eng’g Ethics 1517 (2018).
[14] Maurice E. Stucke & Allen P. Grunes, Big Data and Competition Policy (Oxford Univ. Press 2016).
[15] OECD, Principles on Artificial Intelligence (2021)
[16] Supra at 15
[17] Tim Miller, Explanation in Artificial Intelligence: Insights from the Social Sciences, 267 Artificial Intelligence 1 (2019).
[18] A. Jobin, M. Ienca & E. Vayena, The Global Landscape of AI Ethics Guidelines, 1 Nature Mach. Intelligence 389 (2019)
[19] J. Whittlestone, R. Nyrup, A. Alexandrova, K. Dihal & S. Cave, The Role and Limitations of Ethics in AI, in Proceedings of the 2019 CHI Conference on Human Factors in Computing Systems 1–15 (2019).
[20] C. B. Frey, M. A. Osborne & A. M. Holm, The Future of Employment: How Susceptible Are Jobs to Computerization? , 114 Technological Forecasting and Social Change 254 (2019)
[21] UNESCO, Recommendation on the Ethics of Artificial Intelligence (2021).
[22] Brad Smith & Harry Shum, The Future Computed: Artificial Intelligence and its Role in Society (Microsoft Corp. 2018)
[23] Sundar Pichai, AI at Google: Our Principles, Google AI Blog (2018), https://ai.google/principles/.
[24] Carole Cadwalladr & Emma Graham-Harrison, Revealed: 50 Million Facebook Profiles Harvested for Cambridge Analytica in Major Data Breach, The Guardian (Mar. 17, 2018)
[25] Jack Nicas, Natalie Kitroeff, David Gelles & James Glanz, Boeing’s 737 Max: What’s Happened After the 2 Deadly Crashes, N.Y. Times (Oct. 14, 2019)
Disclaimer: The materials provided herein are intended solely for informational purposes. Accessing or using the site or the materials does not establish an attorney-client relationship. The information presented on this site is not to be construed as legal or professional advice, and it should not be relied upon for such purposes or used as a substitute for advice from a licensed attorney in your state. Additionally, the viewpoint presented by the author is personal.
0 Comments